Publications
An dieser Stelle publizieren wir Informationen zu Studien, welche direkt oder indirekt mit unseren Lösungen oder angewandten Technologien, oder Personen aus unseren Firmen im Zusammenhang stehen.
Es kann sich auch um Publikationen von unseren Kunden handeln, welche unsere Lösungen im Zusammenhang mit einer Studie eingesetzt haben.
Here we publish information on studies that are directly or indirectly related to our solutions or applied technologies, or persons from our companies.
It may also be publications from our customers who have used our solutions in connection with a study.
Latest publication
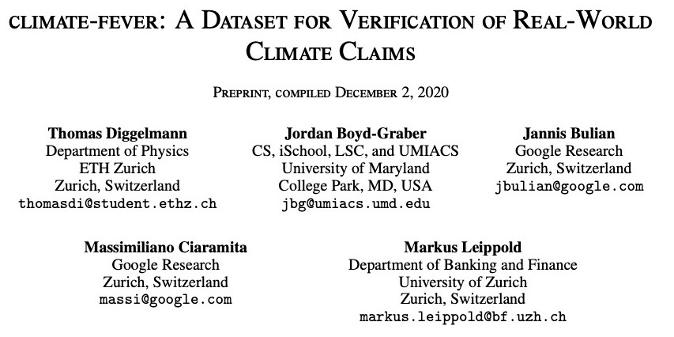
Wir führen «CLIMATE-FEVER» ein, einen neuen öffentlich zugänglichen Datensatz zur Überprüfung von Ansprüchen im Zusammenhang mit dem Klimawandel.
Indem wir der Forschungsgemeinschaft einen Datensatz zur Verfügung stellen, wollen wir die Arbeit an der Verbesserung der Algorithmen für die Suche nach Beweisen für klimaspezifische Behauptungen erleichtern und fördern, die zugrunde liegenden sprachlichen Verständnisprobleme angehen und letztlich dazu beitragen, die Auswirkungen von Fehlinformationen auf den Klimawandel zu mildern. Wir passen die Methodik von FEVER [1], dem größten Datensatz künstlich entworfener Ansprüche, an reale Ansprüche an, die aus dem Internet gesammelt werden.
Während dieses Prozesses konnten wir uns zwar auf die Expertise renommierter Klimawissenschaftler stützen, doch erwies sich dies als keine leichte Aufgabe. Wir diskutieren die überraschende, subtile Komplexität der Modellierung von klimabezogenen Ansprüchen aus der realen Welt innerhalb des \textsc{fever}-Rahmens, die unserer Meinung nach, eine wertvolle Herausforderung für das allgemeine Verständnis der natürlichen Sprache darstellt.
Wir hoffen, dass unsere Arbeit den Beginn einer neuen aufregenden, langfristigen gemeinsamen Anstrengung von Klimawissenschaft und KI-Gemeinschaft markiert.
We introduce CLIMATE-FEVER, a new publicly available dataset for verification of climate change-related claims.
By providing a dataset for the research community, we aim to facilitate and encourage work on improving algorithms for retrieving evidential support for climate-specific claims, addressing the underlying language understanding challenges, and ultimately help alleviate the impact of misinformation on climate change. We adapt the methodology of FEVER [1], the largest dataset of artificially designed claims, to real-life claims collected from the Internet.
While during this process, we could rely on the expertise of renowned climate scientists, it turned out to be no easy task. We discuss the surprising, subtle complexity of modeling real-world climate-related claims within the \textsc{fever} framework, which we believe provides a valuable challenge for general natural language understanding.
We hope that our work will mark the beginning of a new exciting long-term joint effort by the climate science and AI community.
Das Problem mit den Behauptungen:
Fake News, verfremdeter Informationen, nicht belegter Texte, Falschmeldungen bis hin zu Lügen ist allmählich allen Internet Benutzern bekannt und viele Nutzer sind schon betroffen davon.
Die oben genannte und publizierte Studie fokussiert auf das Thema Klima und Klima-Statements. Natürlich kann das Prinzip auf alle anderen Themen angewandt werden.
BrainDogs™ ist eine unserer Anwendungen, welche unterschiedliche Lösungen in den Bereichen der intelligenten Text und Dokumentanalyse anbietet. BrainDogs™ kann ganze Texte, Konzepte oder Ideen verstehen und vergleichen.
The problem with the claims:
Fake news, alienated information, unsupported texts, false reports and even lies are gradually becoming known to all Internet users and many users are already affected by them.
The above mentioned and published study focuses on the topic of climate and climate statements. Of course the principle can be applied to all other topics.
BrainDogs™ is one of our applications, which offers different solutions in the areas of intelligent text and document analysis. BrainDogs™ can understand and compare whole texts, concepts or ideas.
Official document download:
Official publishing site:
Thomas Diggelmann, MSc ETH Physics, ist Mitbegründer und Leiter der Forschung bei ai-one™ Inc. Tomi ist massgeblich an der Entwicklung unserer Algorithmen für unsere verschiedenen Anwendungen beteiligt. Er ist unser Chief AI Strategy
Thomas Diggelmann, MSc ETH Physics, co-Founder and Head of Research at ai-one™ Inc. Tomi is significantly involved in the development of our algorithms for our various applications. He is our Chief AI Strategy
Die Semantische Informationstechnik (only in German available at present)
https://www.springer.com/gp/book/9783658319373#aboutBook
Editors: Ege, Börteçin, Paschke, Adrian (Hrsg.) diverse Autoren, Erscheinungsdatum: Frühjahr 2021
eBook ISBN 978-3-658-31938-0 | DOI 10.1007/978-3-658-31938-0 | Softcover ISBN 978-3-658-31937-3
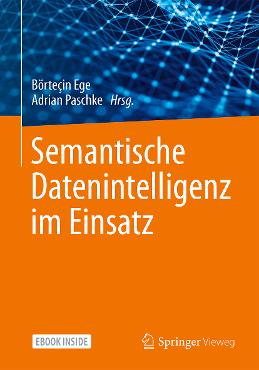
Bedeutung und Anwendungen Semantischer Technologien für die Entwicklung von datenbasierten Systemen wie Neuronale Netze, Deep Learning und Machine Learning.
Semantische Technologien haben mit der Entwicklung von datenbasierten Systemen wie Neuronale Netze, Deep Learning und Machine Learning (ML) ihre Bedeutung nicht verloren, sondern werden als effiziente wissensbasierte Systeme immer wichtiger. Denn intelligente Systeme der Zukunft müssen nicht nur in der Lage sein zu sagen, was sie entschieden haben, sondern auch wie sie zu dieser Entscheidung gekommen sind. Solche Systeme sind jedoch nur mit wissensbasierten Systemen auf der Grundlage von semantischen Technologien erreichbar. Heute reichen die Anwendungen von semantischen Systemen von der semantischen Suche, Knowledge Graphs, Chatbots, NLP in der Medizin bis zur Telekommunikation, Verwaltung und Robotik. Semantische Technologien werden spätestens mit dem Voranschreiten des Internet of Things (IoT) und Industrie 4.0 Anwendungen allgegenwärtig sein. Dies ist unumgänglich, denn ohne sie ist auch die Interoperabilität unter Maschinen und insbesondere unter Roboter für eine intelligente Zusammenarbeit und Produktion nicht so einfach umsetzbar. Dafür gibt es bereits heute zahlreiche Beispiele aus der Industrie.
Kapitel 11: «Semiotik, ein Schlüsselelement für Systeme mit künstlicher Intelligenz»
Autor: Walter Diggelmann, ai-one™
Automatische Systeme, welche mit Hilfe von künstlicher Intelligenz Texte auswerten sind hoch im Kurs, und es werden immer mehr. Diese Systeme versuchen eine Botschaft in einem Text zu verstehen und in einen Zusammenhang zu stellen. Es wird darüber hinaus auch versucht festzustellen, ob die Botschaft eine negative, positive oder neutrale Aussage zu einer Sache, einer Aktion oder einem Thema etc. macht. Eine Nachricht oder Information kann auf sehr unterschiedliche Weise gehört und verstanden werden. Sie kann aus verschiedenen Perspektiven gehört werden. Der Empfänger fragt sich wie der Sachverhalt zu verstehen ist, aber auch was der Sender mit der Nachricht erreichen will. Die Antwort, die der Empfänger sich selber gibt, muss allerdings keinesfalls mit der beabsichtigten Bedeutung des Senders übereinstimmen. Um Missverständnisse und Unklarheiten zu vermeiden, müssen die Zusammenhänge der Kommunikation verdeutlicht werden.
Damit Semantische Textanalysen, welche die Grundlage bilden für alle ML-, IoT- und AI- Lösungen optimal funktionieren, ist es wichtig, dass diese für zwei wesentliche Herausforderungen der Textanalyse eine dynamische Lösung bieten.
Kongruente Sätze und Formulierungen aus unterschiedlichen Zeiten und Orten, können inhaltlich eine ganz andere Aussage, Botschaft verkörpern, obwohl sie syntaktisch übereinstimmen.
Hans-Georg Gadamer beschreibt in seiner Theorie «Wahrheit und Methode» einen hermeneutischen Zirkel: «Das Ganze muss aus dem Einzelnen und das Einzelne aus dem Ganzen heraus verstanden werden». Somit enthält der hermeneutische Zirkel ein Paradoxon: Das, was verstanden werden soll, muss schon vorher verstanden worden sein, oder zumindest in Teilen bekannt sein.
Walter Diggelmann erklärt in Kapitel 11, wie das Konzept des semantischen Fingerprints als Lösung für diese Herausforderungen eingesetzt werden kann.
Der semantische Fingerprint basiert auf der Erkenntnis der intrinsischen oder inhärenten Semantik. Das ist die Semantik oder Botschaft eines Texts, wenn er nicht gelesen wird.
Der semantische Fingerprint kann darüber hinaus als Grundlage für sämtliche weiteren linguistischen und statistischen Analysen eingesetzt werden.
NASA - Marshall Space Flight Center Research and Technology Report 2015
https://ntrs.nasa.gov/citations/20160006403
The investments in technology development we made in 2015 not only support the Agency's current missions, but they will also enable new missions. Some of these projects will allow us to develop an in-space architecture for human space exploration; Marshall employees are developing and testing cutting-edge propulsion solutions that will propel humans in-space and land them on Mars. Others are working on technologies that could support a deep space habitat, which will be critical to enable humans to live and work in deep space and on other worlds. Still others are maturing technologies that will help new scientific instruments study the outer edge of the universe-instruments that will provide valuable information as we seek to explore the outer planets and search for life.
Document ID 20160006403
Report Number M16-5259 | NASA/TM-2016-218221
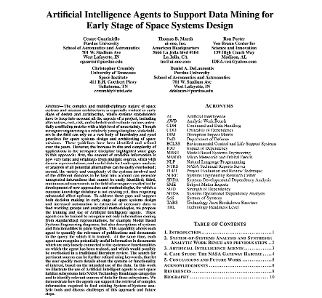
Artificial Intelligence Agents to Support Data Mining for Early Stage of Space Systems Design
978-1-7281-2734-7/20/ ©2020 IEEE
The complex and multidisciplinary nature of space systems and mission architectures is especially evident in early stage of design and architecting, where systems stakeholders have to keep into account all the aspects of a project, including alternatives, cost, risk, and schedule and evaluate various potentially conflicting metrics with a high level of uncertainty. Though aerospace engineering is a relatively young discipline, stakeholders in the field can rely on a vast body of knowledge and good practices for space systems design and architecting of space missions. These guidelines have been identified and refined over the years.
However, the increase in size and complexity of applications in the aerospace discipline highlighted some gaps in this approach: first, the amount of available information is now very large and originates from multiple sources, often with diverse representations, and useful data for trade space analysis or analysis of all potential alternatives can be easily overlooked.
Second, the variety and complexity of the systems involved and of the different domains to be kept into account can generate unexpected interactions that cannot be easily identified; third, continuous advancements in the field of aerospace resulted in the development of new approaches and methodologies, for which a common knowledge database is not existing yet, thus requiring substantial effort upfront.
To address these gaps and support both decision making in early stage of space systems design and increased automation in extraction of necessary data to feed working groups and analytical methodologies, we propose the training and use of Artificial Intelligence agents. These agents can be trained to recognize not only information coming from standardized representations, for example Model Based Systems Engineering diagrams, but also descriptions of systems and functionalities in plain English.
This capability allows each agent to quantify the relevance of publications and documents to the query for which it is trained. At the same time, each agent can recognize potentially useful information in documents which are only loosely connected to the systems or functionalities on which the agent has been trained, and which would possibly be overlooked in a traditional literature review. The search for pertinent sources can be further refined using keywords, that let the user specify more details about the systems or functionality of interest, based on the intended use of the data. In this work we illustrate the use of Artificial Intelligent agents to sort space habitat subsystems into NASA Technology Roadmaps categories and to identify relevant sources of data for these subsystems. We demonstrate how the agents can support the retrieval of complex information required to feed existing System-of-Systems analytic tools and discuss challenges of this approach and future steps.
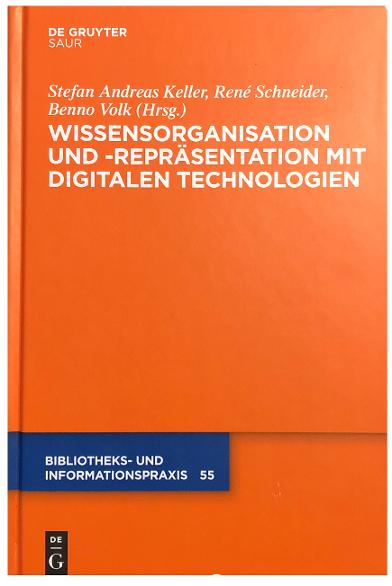
Wissensorganisation und -repräsentation mit digitalen Technologien
https://www.degruyter.com/view/product/205460
Hrsg. v. Keller, Stefan Andreas / Schneider, René / Volk, Benno / Walter Diggelmann (Page 128 - 145)
Im Sammelband werden die sehr unterschiedlichen konzeptionellen und technologischen Verfahren zur Modellierung und digitalen Repräsentation von Wissen in Wissensorganisationen (Hochschulen, Forschungseinrichtungen und Bildungsinstitutionen) sowie in Unternehmen anhand von praxisorientierten Beispielen in einer Zusammenschau vorgestellt. Dabei werden sowohl grundlegende Modelle der Organisation von Wissen als auch technische Umsetzungsmöglichkeiten sowie deren Grenzen und Schwierigkeiten in der Praxis insbesondere in den Bereichen der Wissensrepräsentation und des Semantic Web ausgelotet. Good practice Beispiele und erfolgreiche Anwendungsszenarien aus der Praxis bieten dem Leser einen Wissensspeicher sowie eine Anleitung zur Realisierung von eigenen Vorhaben.Folgende Themenfelder werden in den Beiträgen behandelt: Hypertextbasiertes Wissensmanagement, digitale Optimierung der erprobten analogen Technologie des Zettelkastens, innovative Wissensorganisation mittels Social Media, Suchprozessvisualisierung für Digitale Bibliotheken, semantische Event- und Wissensvisualisierung, ontologische Mindmaps und Wissenslandkarten, intelligente semantische Wissensverarbeitungssysteme, Grundlagen der computergestützten Wissensorganisation und -integration, das Konzept von Mega-Regions zur Unterstützung von Suchprozessen und zum Management von Printpublikationen in Bibliotheken, automatisierte Kodierung medizinischer Diagnosen sowie Beiträge zum Records Management zur Modellbildung und Bearbeitung von Geschäftsprozessen.
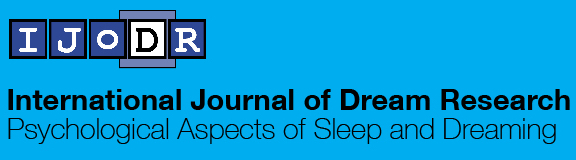
Dream content analysis using Artificial Intelligence
https://journals.ub.uni-heidelberg.de/index.php/IJoDR/article/view/48744
Patrick McNamara (Author), Boston University School of Medicine. Dream content analysis using Artificial Intelligence from ai-one. A dream content analysis system based on A.I. We conclude that the AI-based algorithm developed is a promising tool for detailed analyses of dream content patterns. Kevin Duffy-Deno, Tom Marsh, ai-one Inc. and Thomas Marsh Jr., Boulder Equity Analytics
We developed a dream content analysis system (DCAS) based on an artificial intelligence (AI) algorithm that was trained using a relatively large corpus of over 35,000 dreams. This sample of dreams were supplied by 424 female and 211 male users over 4 years who had posted them at the dream posting website and app Dreamboard.com. Building upon previous dream content ontologies developed by Hall, Van de Castle, Domhoff and Bulkeley, forty-seven reliably identified dream themes emerged from repeated application of algorithm and agent training procedures. DCAS reproduced most of the key dream content themes from these previous ontologies but also returned some unexpected findings. Mixed-model estimation detected significant male-female content differences for 34 dream themes, with female dreams evidencing higher incidence percentages for most themes, but effect sizes were small. Mixed-model logistic regression identified those themes that best predicted self-reported positive or negative mood associated with dreams. We conclude that the AI-based DCAS algorithm developed here is a promising tool for detailed analyses of dream content patterns.
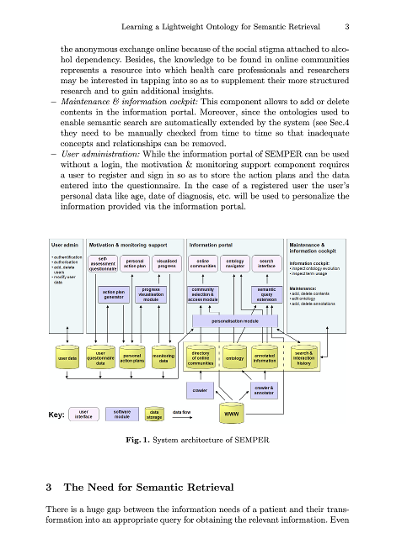
Learning a Lightweight Ontology for Semantic Retrieval in Patient-Centered Information Systems
https://www.igi-global.com/gateway/issue/47946
Ulrich Reimer (University of Applied Sciences St. Gallen, Switzerland), Edith Maier (University of Applied Sciences St. Gallen, Switzerland), Stephan Streit (University of Applied Sciences St. Gallen, Switzerland), Thomas Diggelmann (ai-one, Switzerland)
The paper introduces a web-based eHealth platform currently being developed that will assist patients with certain chronic diseases. The ultimate aim is behavioral change. This is supported by online assessment and feedback which visualizes actual behavior in relation to target behavior. Disease-specific information is provided through an information portal that utilizes lightweight ontologies (associative networks) in combination with text mining. The paper argues that classical word-based information retrieval is often not sufficient for providing patients with relevant information, but that their information needs are better addressed by concept-based retrieval. The focus of the paper is on the semantic retrieval component and the learning of a lightweight ontology from text documents, which is achieved by using a biologically inspired neural network. The paper concludes with preliminary results of the evaluation of the proposed approach in comparison with traditional approaches.
Copyright © by DIWA-Capital 2013-2021, info@diwa-capital.com | SWISS MADE |